What is This?
Data aggregation is the process of gathering raw data and summarizing it to make meaning. This is a necessary process in research and inherently introduces researcher bias. The decisions that a researcher makes to decide how to summarize their data lead to the inclusion or exclusion of groups. Researchers need to be intentional in their summary choices, particularly when it comes to grouping people to minimize the bias introduced. This is a form of aggregation bias.
For example, a researcher is seeking to understand the relationship between household income and the average number of years of education within the household. They gather data from four cities and plot this data to find the line of best fit. In so doing they find a strongly positive correlation between average years of education and household income. They conclude that more years of education is strongly positively correlated with household income. However, they have aggregated all four cities into one analysis instead of looking at them separately. Grouping by city reveals that in two of the four cities, there is actually a negative correlation between average education and household income.
Often, due to small sample sizes, underrepresented and minority groups are aggregated into larger groups for analyses. It is important in QuantCrit and equity-based research to disaggregate groups based on race/gender and other identifiers that have been systemically and systematically oppressed in the societies we study.
The Fine Balance of Data Aggregation
While aggregating is necessary to achieve large enough sample sizes that regression outputs and interpretations are reliable, aggregation can also hide important voices that would signal change is needed.
Recommendations:
1. Know the limits of the tools you are using. If the package or estimator you are using to analyze your dataset require a minimum sample size, be aware of that minimum and use it to maximal effect. For instance, in one of our analyses we examined over 15,000 student outcomes on the Force Concept Inventory from the LASSO database. We could have looked at how all 15,000 of those students are doing but we would have missed important data from different groups of interest. White men dominate our dataset, as is the case for STEM in general. So, by looking at the entire set of 15,000 cases, we would have primarily been seeing the average student’s performance – a white man. Depending on the analysis we’re doing our statistical tools required a minimum sub-group sample size that can range from less than 20 to over 200 cases. We identified each group that met that minimum threshold and disaggregated the ten race/gender groups we could study. This not only allowed us to more accurately represent the data, but we could then identify educational debts owed to marginalized groups. We still fully understood the performance of white males, but we gained a deeper understanding of all the groups we studied.
2. Be careful about your analysis! If you do not disaggregate your data, you cannot say that your findings are generalizable to all groups – only to the dominant group in your sample. There were many groups we did not study because their population in the dataset did not meet the sample size cutoff. We have been cautious about not making any claims about those groups or being so vague in our findings as to inadvertently include them.
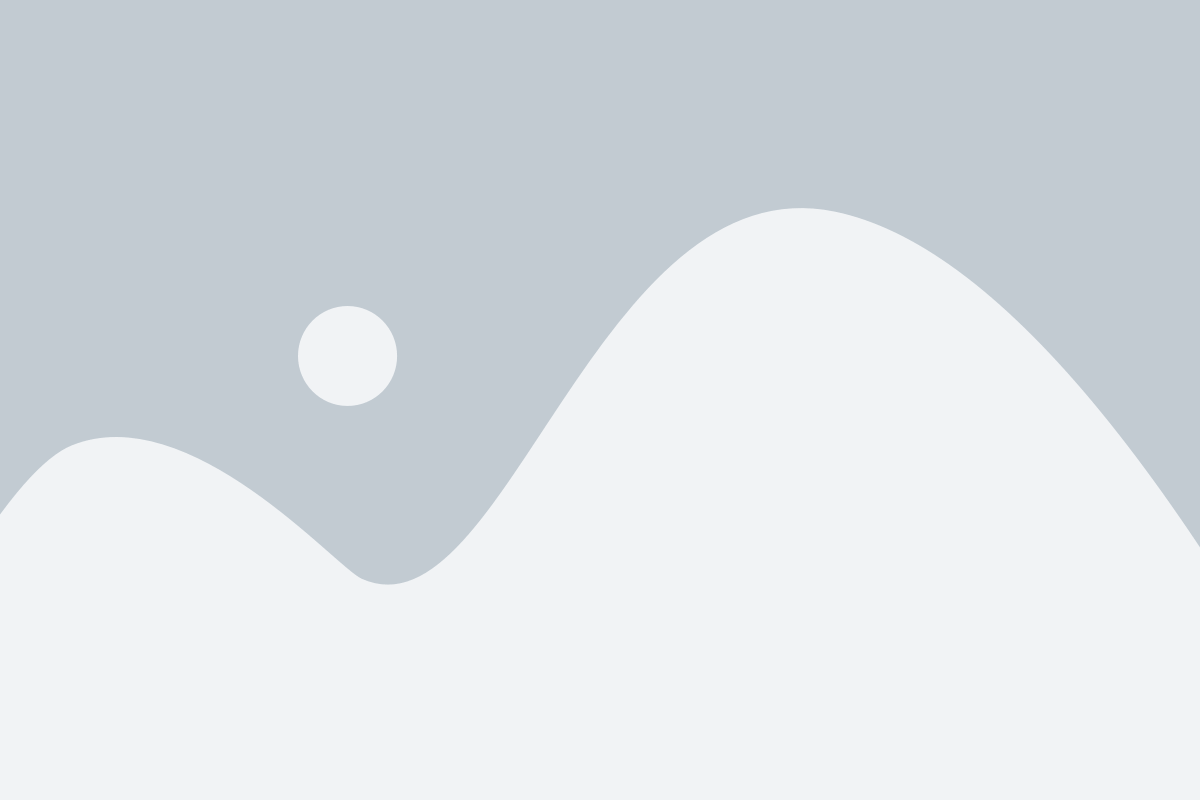
There are a number of articles that address this fine balance between achieving the sample size necessary for the tools used and also disaggregating to represent as many groups as possible. See below for recommended readings:
- Gillborn, D., Warmington, P., & Demack, S. (2018). QuantCrit: education, policy,‘Big Data’and principles for a critical race theory of statistics. Race Ethnicity and Education, 21(2), 158-179
- Shafer, D., Mahmood, M. S., & Stelzer, T. (2021). Impact of broad categorization on statistical results: How underrepresented minority designation can mask the struggles of both Asian American and African American students. Physical Review Physics Education Research, 17(1), 010113.
- Van Dusen, B., & Nissen, J. (2020). Equity in college physics student learning: A critical quantitative intersectionality investigation. Journal of Research in Science Teaching, 57(1), 33-57.
- Traxler, A., Henderson, R., Stewart, J., Stewart, G., Papak, A., & Lindell, R. (2018). Gender fairness within the force concept inventory. Physical Review Physics Education Research, 14(1), 010103.