Missing Data
Importance in education research
DBER datasets are typically incomplete. For example, when administering a concept inventory, some students will miss the pretest and/or the posttest. Prior to developing a regression model, a dataset must be made complete. In DBER, this has typically been done by removing data from students who have partial data in a process called complete case analysis (Nissen, Donatello, & Van Dusen, 2019). In data science, missing data is often addressed through a form of multiple imputation. In a simulation study, Nissen, Donatello, & Van Dusen (2019) demonstrated that complete case analysis led to more biased findings than multiple imputation when analyzing DBER concept inventory data but did not examine issues of equity.
Equity issue
Data are most likely to be missing from students who earn lower grades in a course (Nissen, Donatello, & Van Dusen, 2019). Performing complete case analysis has a dual impact on equity analyses by (1) preferentially removing data from URM students and (2) restricting the span of data examined. This will limit power and likely make differences in performance across groups appear artificially small.
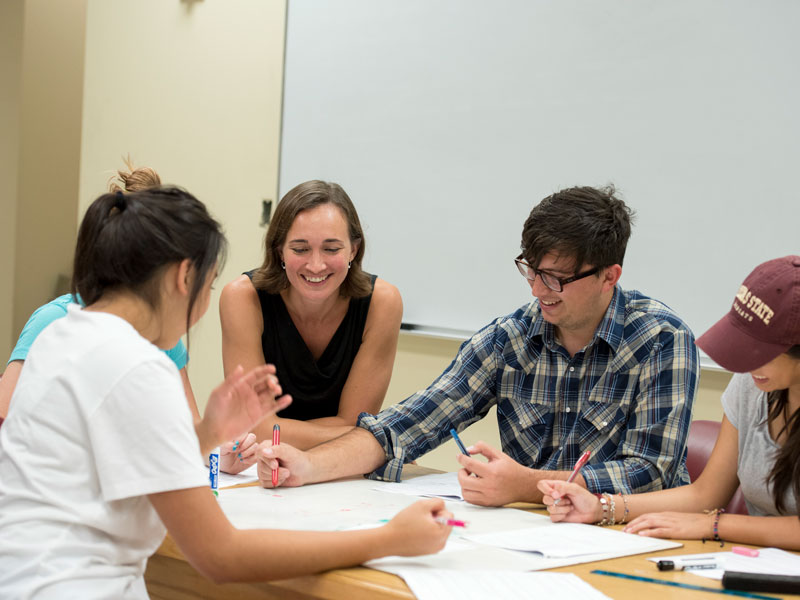
SUPPORT STEM EQUITY
STEM Equity is continuously adding to our personal and professional resources and partners in the mission of equitable STEM education.
If you know of an organization we should know about or partner with, or would like to support STEM Equity’s mission, please contact us.