Model uncertainty
Importance in education research
Model uncertainty is a measure of how well a model fits a dataset and is often expressed in terms such as standard errors. Researchers use these measures to inform how confident they are in the results from a model. In DBER, the most common method for expressing confidence is through the use of p-values as go/no-go tests that determine if an effect exists. The American Statistics Association has recommended against this misuse of p-values for understanding model uncertainty as it can lead to false claims (Wasserstein & Lazar, 2016; Wasserstein et al., 2019).
Equity issue
When investigating issues of equity for URM students, it can be difficult to collect datasets with sufficient power to prevent the inaccurate dismissal of inequities due to having p-values greater than 0.05 (a false negative error). Other methods for conveying model uncertainty, such as confidence intervals, allows for the more nuanced reporting of estimated inequities alongside the range of potential values.
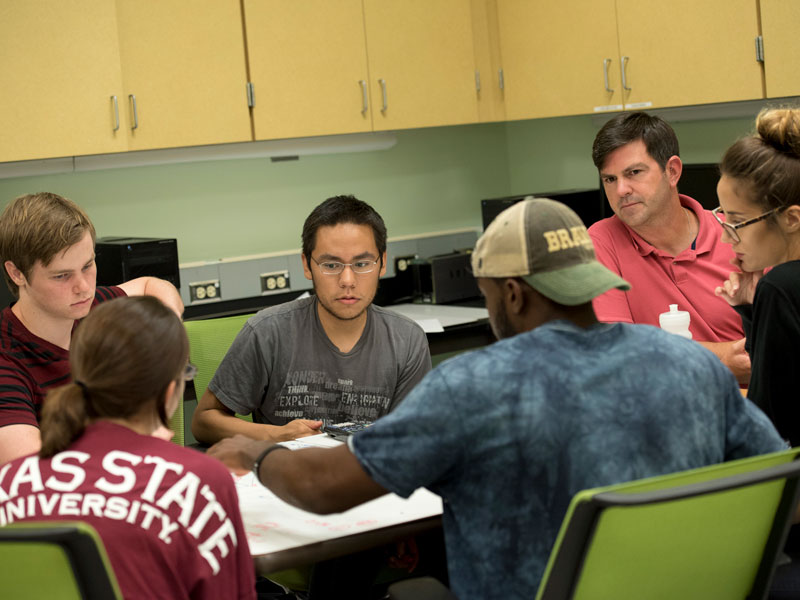
SUPPORT STEM EQUITY
STEM Equity is continuously adding to our personal and professional resources and partners in the mission of equitable STEM education.
If you know of an organization we should know about or partner with, or would like to support STEM Equity’s mission, please contact us.