DAta cleaning
Importance in education research
Data cleaning is performed prior to analysis to remove spurious data from a dataset (Osborne & Overbay, 2008). There is a tension between maximizing the removal of spurious or unreliable data and minimizing the removal of accurate data. If a data cleaning technique systematically leaves spurious data or removes accurate data, it can bias findings.
Equity issue
Any data cleaning technique (including doing no cleaning) has the potential to have differential impacts of data across demographic groups and bias equity findings. For example, if a researcher follows the recommendation of Coletta & Steinert (2020) and removes the data for students who have pretest scores over 80%, then they are selectively removing data from students with the strongest physics backgrounds. As Van Dusen & Nissen (2019a) showed, these students are most likely to be white men. In high performing classes, this data cleaning technique will likely make differences in performance across groups appear artificially small.
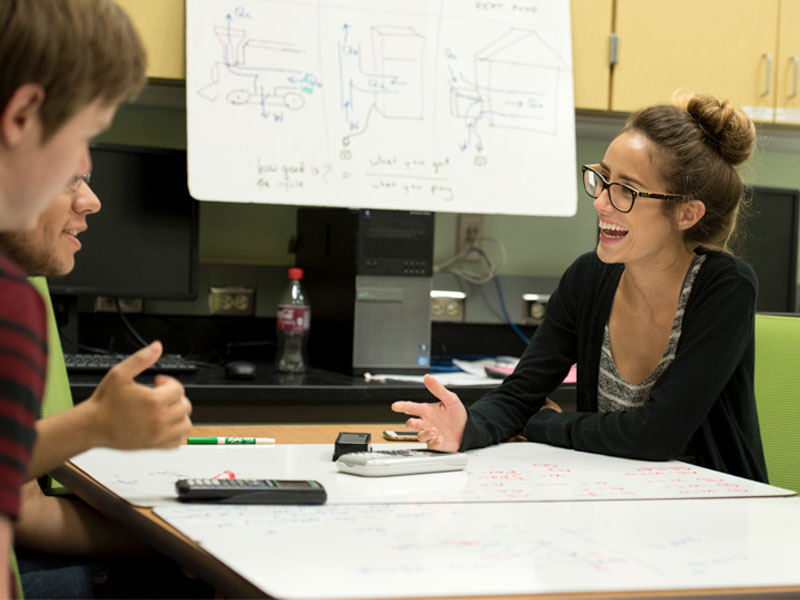
SUPPORT STEM EQUITY
STEM Equity is continuously adding to our personal and professional resources and partners in the mission of equitable STEM education.
If you know of an organization we should know about or partner with, or would like to support STEM Equity’s mission, please contact us.