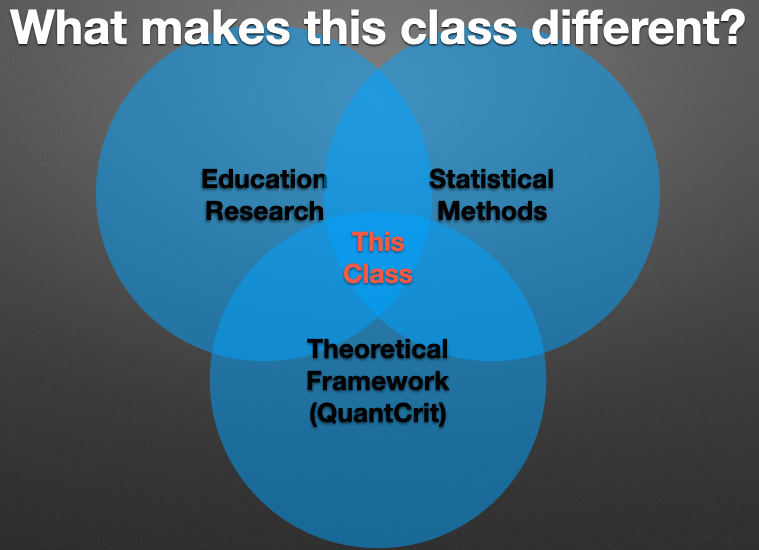
This semester I set out to translate our team’s thinking about and research into using QuantCrit perspective to inform research into a course for PhD students. Not knowing of any other QuantCrit methods curriculums, I began working from scratch. Teaching the class has been a highly enjoyable and I thank my amazing students for engaging so fully in the intellectual work. I hope to refine my curriculum and make it publicly available for others who wish to teach a similar course. But that will take time and I wanted to share an overview of the course (so far) for those who might be interested:
Week 1: Overview of the course, create groups for the semester, and identify a dataset for each group to use throughout the semester. It’s critical that the datasets have student social identifier information (e.g., race and gender) so that they can answer research questions about diversity, equity, and inclusion.
Week 2: Get to know their datasets. Where did they come from and what biases might already be baked into them? Brainstorming research questions. We include an overview of QuantCrit.
Week 3: Creating descriptive statistics and basic figures. Identifying trends that are important to answering their research questions.
Week 4: Data cleaning. What kinds of bad data might exist in your dataset? How might removing data bias your findings? How might not removing data bias your findings?
Week 5: Missing data. Who are missing in your data? What causes may have led them to be missing? How might this bias your findings?
Week 6: Multiple imputation. How can we create multiple imputation models that will limit the biases due to missing data? Students also read and critique a pair of articles that both examine issues of DEI, but one from a QuantCrit perspective and one from a deficit perspective.
Week 7: Creating regression models. How do the variables we include in our models influence what the models are saying? For example, if prior preparation is included in a model, the model will now predict how groups will do if they has the same prior preparation. This is something that is not usually true. We also discuss what it mean to include a social identity variable in a model. It’s important that we consider how to interpret these variables in ways that don’t support deficit ways of thinking. For example, we name racism and sexism as causes, rather than race and gender.
Week 8: Checking model assumptions and multi-level models.
Week 9: Model specification and defining equity. How might the model specification process biased findings? For example, if p-values were used to determine if variables should be included in a model, then it is highly unlikely that interaction terms for minoritized groups will be included.
—– This is as far as I am at the moment, but I’m including a rough plan for the rest of the course.
Week 10: Data visualization. How to show data in ways that don’t support deficit interpretations.
Week 11: P-values and their pernicious impacts, particularly in diversity, equity, and inclusion investigations.
Week 12: More on uncertainty and effect sizes.
Week 13-15: Working on their analysis, reading and critiquing examples of quantitative diversity, equity, and inclusion research.
Final: Submit their draft of a QuantCrit paper based on their analysis (minus the literature review).